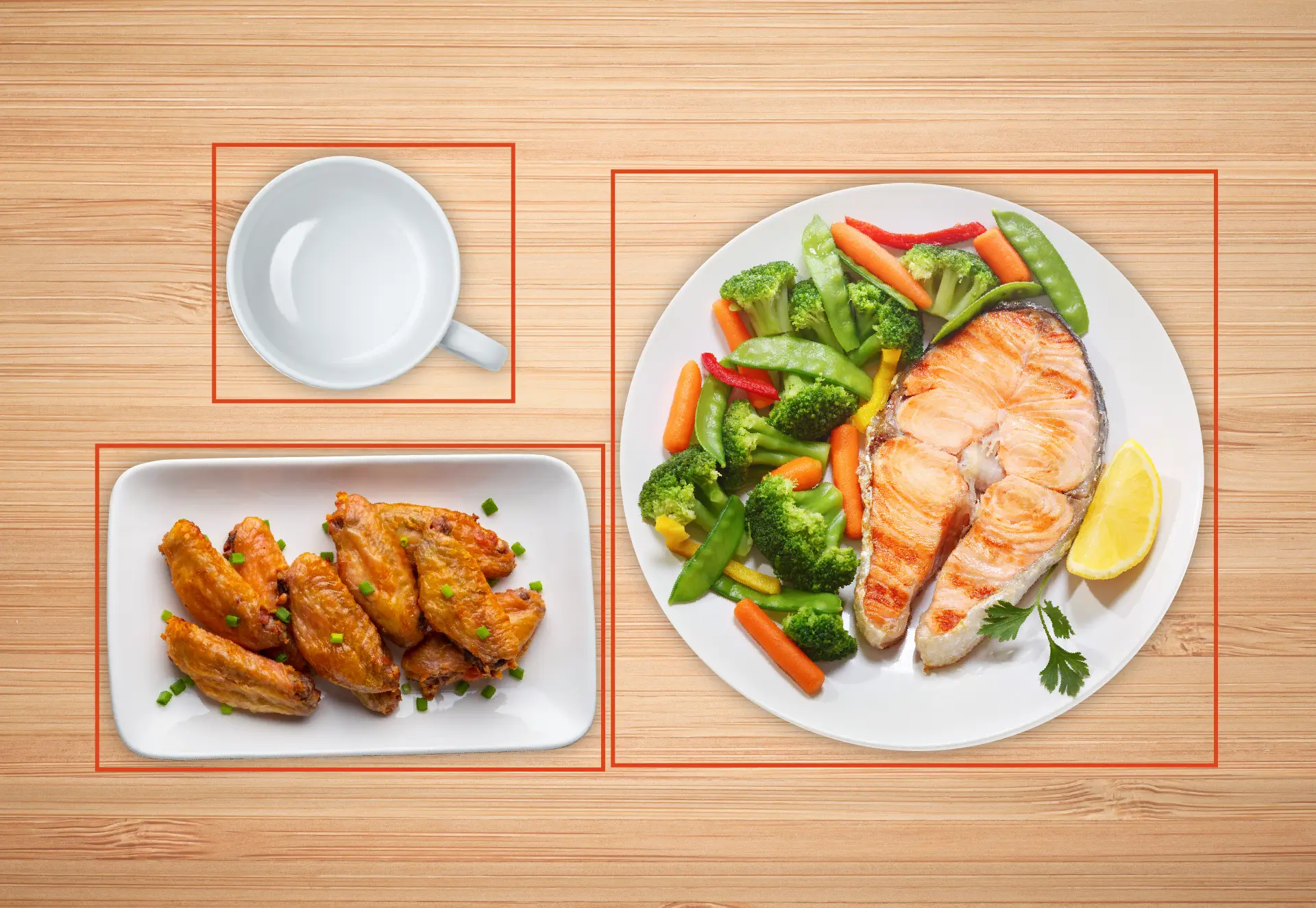
Real-World Challenges in AI Food Recognition (Part 1)
May 20, 2025
With the rapid advancement of AI, more and more food businesses are experimenting with image recognition technology to improve their operations. Whether it’s to help staff take orders, verify meals before serving, or enable self-checkout for customers, AI-powered food recognition is being used in a variety of ways—all aiming for the same goal: to identify food items faster and more accurately.
Surprisingly, though, food recognition is much more complex than it looks. Especially in common settings like cafeterias, bento shops, or Japanese restaurants, even the most ordinary-looking meals can present unexpected challenges for AI.
In this article, we’ll walk you through four real-world cases encountered by Viscovery, an AI image recognition solution provider, to explore the practical challenges of using AI for food recognition in the food service industry—and how these challenges have been overcome. These technologies are now successfully used in places like hospital cafeterias and “Hi Noodle,” a brand under Haidilao Singapore. By letting computers take on the task of recognizing meals, businesses can streamline operations and boost efficiency.
Read More: AI Revolutionizes Haidilao “Hi Noodle” Self-Checkout Experience
Bento Boxes: Every Compartment Counts
Many bento boxes are designed with multiple compartments, each holding a different dish—like a main course, some veggies, or a marinated egg. Sounds easy to recognize, right?
Not quite.
Humans can tell what’s what by looking at color, shape, and placement. But for AI, the first step is figuring out where the compartments are—only then can it identify what’s inside each one. When ingredients overflow or sauces spill across dividers, the recognition process becomes even more complicated.
Viscovery’s robust AI models have been trained to handle these scenarios. By balancing compartment detection with dish identification, the system achieves consistent recognition performance across various bento box designs.
Read More: How is AI Used in the Bento Industry
Read More: Taiwan’s Bento Market is Booming: How Can AI Help Businesses Stay Competitive?
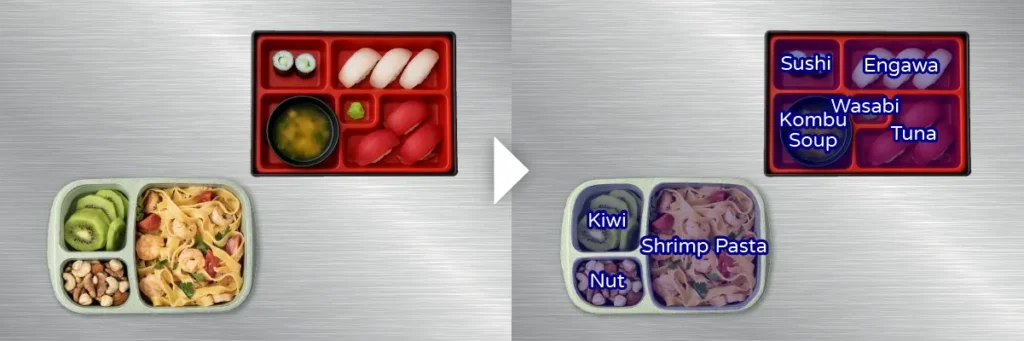
Mixed Plates: No Dividers? No Problem
Unlike bento boxes, many meals are served on a single plate with no clear separation between items—think of buffet plates, sizzling platters, or cafeteria trays. These dishes often have multiple items mixed together on one plate.
In these cases, AI must learn to visually distinguish between individual dishes on the same plate.
This becomes tricky when different foods are similar in color, placed close together, or partially covered in sauces. For instance, a mix of stir-fried noodles and braised cabbage or curry rice with chicken nuggets can easily confuse the system.
To tackle this, Viscovery trains its models with large sets of annotated food images (known as datasets) to improve recognition precision in these visually complex scenarios.
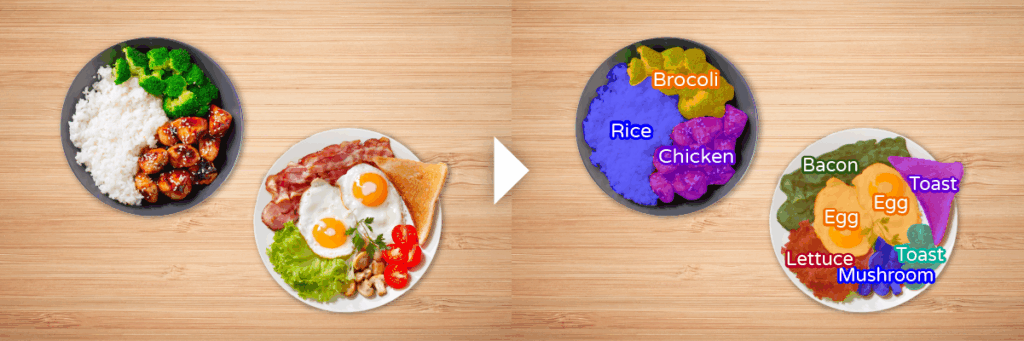
Skewers and Fried Snacks Recognition
Let’s move on to something you’d often find at night markets or izakayas: skewers and fried items.
Imagine a plate with a dozen skewers—how does AI tell them apart and count each one correctly? Traditional systems might see the entire bunch as one item, or even mistake the sticks for food.
Viscovery’s next-gen recognition system can now distinguish individual skewers and accurately identify which parts are edible and which are just serving tools like skewers. It also avoids misreading shadows between items as food, which significantly improves recognition accuracy.
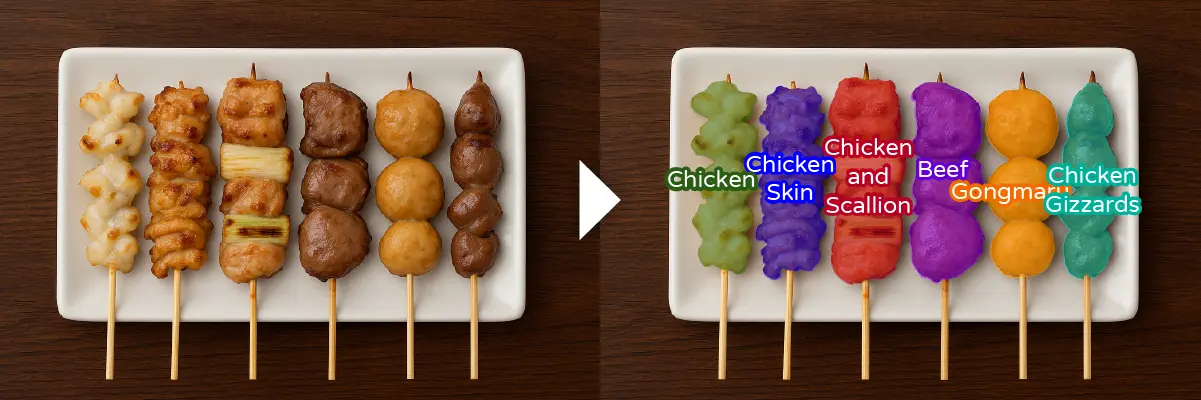
Sushi: How Many Pieces? How Many Types?
Sushi is often arranged in a colorful mix of different types, placed closely together. While it looks visually appealing to us, it poses a serious recognition challenge for AI.
Compared to bentos or skewers, sushi poses a dual challenge: recognizing a wide variety of items (like salmon, tamago, or futomaki) and correctly counting how many of each type there are. For example: 2 pieces of salmon, 1 octopus, 1 tuna… If the system miscounts, it could impact order confirmation or pricing.
The shiny surfaces of trays, sauce spills, and overlapping items make recognition even trickier. But thanks to Viscovery’s proprietary tools, the AI keeps learning from new images in real-world use—getting better with every scan.
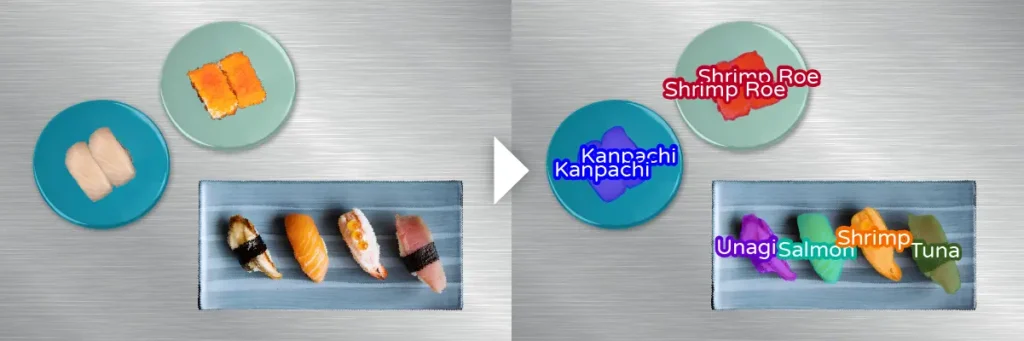
AI Food Recognition: Simpler Than Ever—But Still a Challenge
From bentos and cafeteria trays to skewers and sushi, teaching AI to understand what it sees and how much is there is no small feat. It’s not just image recognition—it’s about making sense of food that’s messy, overlapping, and always looking a little different in the real world.
The good news? These challenges are being solved. More and more restaurants, noodle shops, and corporate cafeterias are now using AI to speed up checkout, reduce human error, and assist kitchens in verifying meal prep—helping to optimize the entire workflow.
In our next article, we’ll explore a new twist: what happens when AI shifts from recognizing food… to recognizing dishes and tableware?