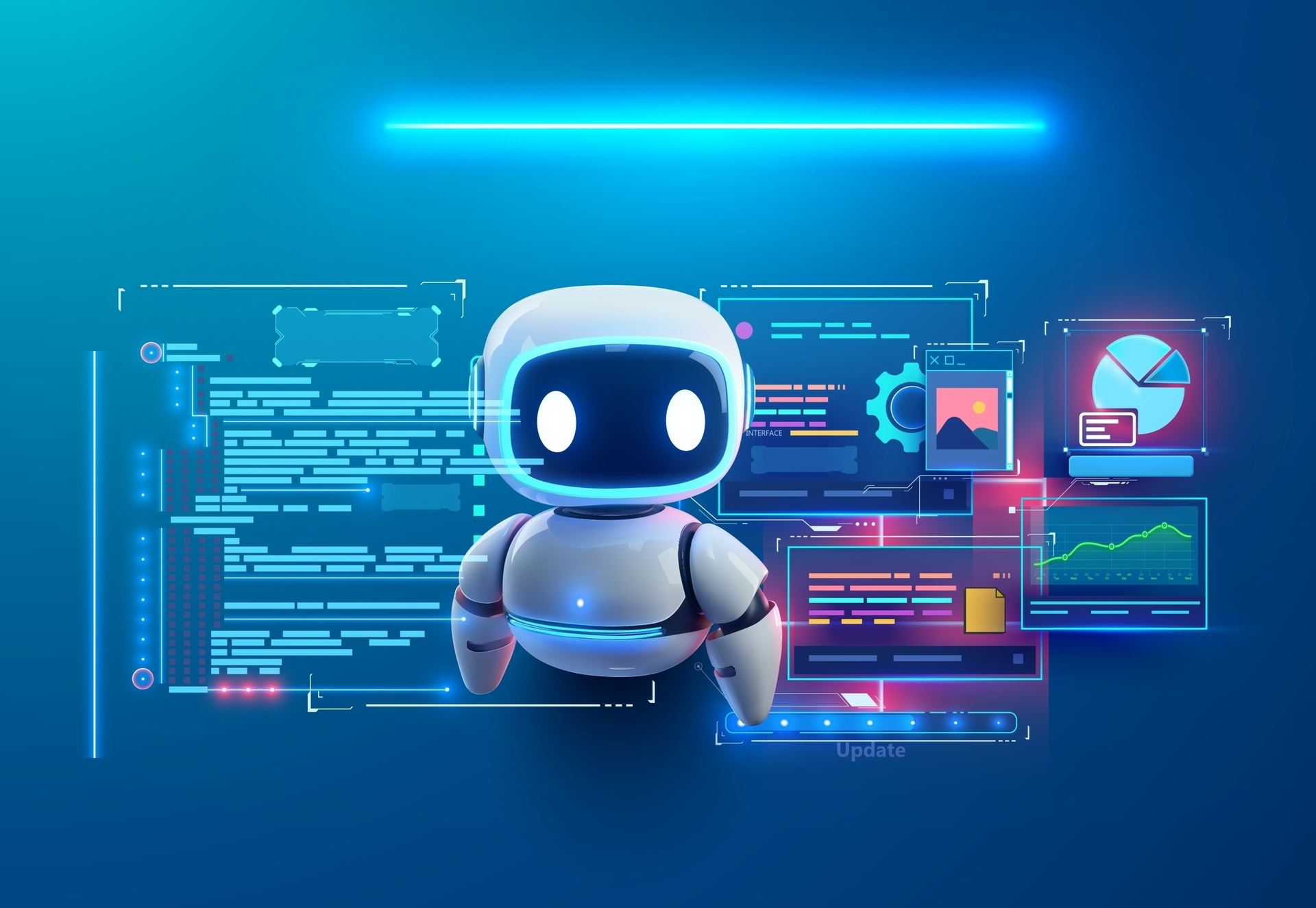
AI Agentic Workflow: A New Era of Smarter, More Collaborative AI
August 29, 2024
As technologies like Chatbots and GPT have matured, people are beginning to think about how to make AI even more advanced. The goal is to move beyond the one-command, one-action approach and allow AI to self-assess its generated content, refine its responses, and ultimately deliver the highest-quality answers by eliminating errors and iterating on its output.
There is also a growing expectation that AI systems can communicate, balance each other out, and collaborate—essentially forming an AI team capable of autonomously executing complex tasks and solving intricate problems. This would result in more refined AI-generated outputs and, ultimately, help us achieve our goals. This vision has given rise to the concept of the AI Agentic Workflow, one of the most discussed topics in recent tech circles.
A Smarter, More Sophisticated AI
When using text generation tools like ChatGPT or Gemini, or various online image generation tools, we have learned not to expect a perfect response from AI on the first try, especially for more complex questions. After receiving the initial response, we usually provide feedback, guiding the AI to produce an answer that better aligns with our expectations.
While the quality of the prompt plays a significant role—the clearer and more specific the prompt, the better the AI understands our needs—modifications are often unavoidable.
For example, if we ask an AI to generate an article about future technologies, the first draft might lack depth or miss key points. In response, we can instruct the AI to elaborate on certain sections or reframe specific ideas. With our feedback, the AI will refine its output, gradually bringing it closer to what we envision.
What is an AI Agent?
Before diving deeper into AI Agentic Workflows, let us define what an AI agent is. Simply put, an AI agent can be thought of as a “human proxy.” It simulates human thought and behavior to make decisions within a given scope and can even communicate and collaborate with other AI systems. The ultimate goal is to deliver a high-quality output that has been thoroughly revised and perfected.
Andrew Ng, one of AI’s most influential figures, discussed four design patterns for AI Agentic Workflows during a lecture1 :
- Reflection
- Tool Use
- Planning
- Multiagent Collaboration
Ng even noted that GPT-3, when using an Agentic Workflow, performed better than GPT-4 which hadn’t yet adopted this approach—showing how effective the Agentic Workflow can be in AI systems.
Reflection: Optimizing Outputs Through Self-Assessment
Reflection is one of the most widely applied design patterns in AI Agentic Workflows. It enables AI to self-assess and revise its outputs. Like the example mentioned earlier, when we ask an AI to generate an article on future technologies, an AI with the “Reflection” design pattern will continuously check its output. Rather than rushing to provide the first version, it will iteratively refine the content until it reaches a more satisfactory result.
This self-assessment process greatly improves the accuracy and reliability of AI, leading to its widespread adoption as a standard feature in most AI-powered technologies today.
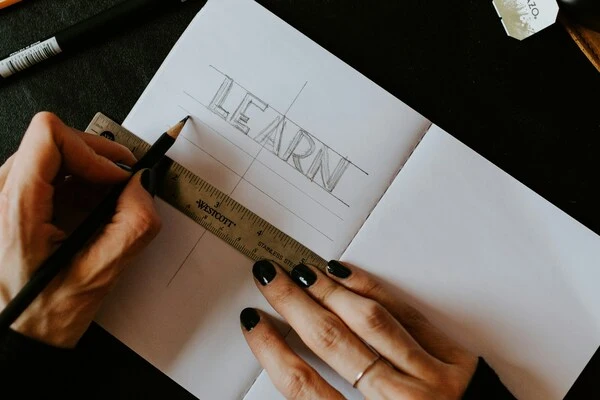
Tool Use: Expanding AI’s Capabilities
Without external resources and tools, AI systems like GPT cannot directly search the web, run code, or process images. To overcome these limitations, developers have added a “Tool Use” mode, enabling AI to perform tasks beyond its core capabilities. For example, if you ask an AI for the latest information on a specific topic, it can use a web search tool to provide real-time updates.
Tool Use makes AI more versatile. Large Language Models (LLMs), for instance, are no longer limited to basic text generation tasks. Instead, they can perform a wide range of operations, continually expanding their abilities and allowing AI to be effective in a broader range of scenarios.
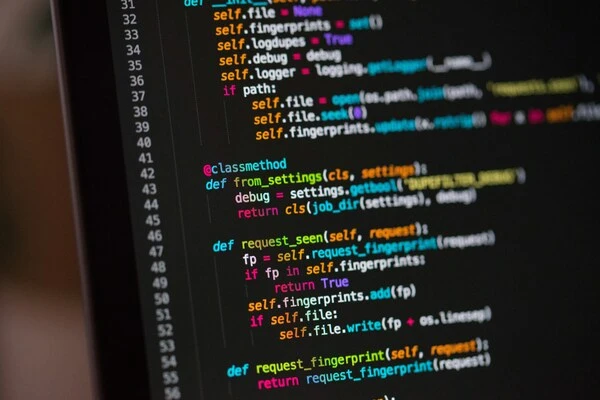
Planning: Breaking Down Complex Tasks Step by Step
Planning is another crucial design pattern that allows AI agents to break down complex tasks and execute them step by step. This approach is especially useful in scenarios that require multi-step operations.
Consider an example where an AI-powered drone is tasked with delivering a package through a complex environment, such as a forest. The mission might involve several steps, requiring the drone to make real-time decisions when facing various challenges. Here’s how the planning might look: route planning, obstacle avoidance, battery management, weather adaptation, and delivery execution.
First, the AI drone needs to plan the best route from start to finish, ensuring both safety and efficiency. It also needs to have an obstacle avoidance plan—what happens if a barrier suddenly appears, like a fallen tree blocking the path? The drone might choose to reroute or increase altitude to avoid the obstacle. It must also monitor its battery level in real-time and have a contingency plan for low power, such as identifying the nearest safe landing spot. Bad weather is another unpredictable factor; the drone must be able to assess weather conditions and decide when to land or continue flying. Finally, there is the delivery execution plan: determining how high the drone should be when dropping off the package and whether someone needs to be present to receive it.
While the “Planning” design pattern is still in development, it has already shown impressive results in certain use cases.
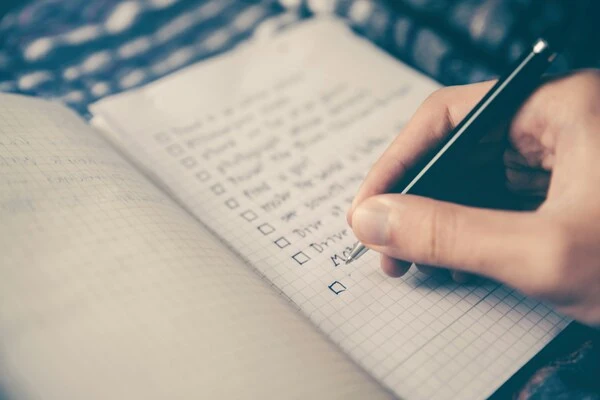
Multiagent Collaboration: Enhancing Task Efficiency Through Teamwork
Multiagent Collaboration involves multiple AI agents working together, each taking on different roles to complete a task, functioning much like a small AI team.
One fascinating application of multiagent collaboration was highlighted in The Batch, an AI newsletter from DeepLearning.AI, founded by Andrew Ng—specifically, the use of multiple agents to translate literary works. 2
As mentioned earlier, Large Language Models (LLMs) are primarily used for text-related tasks, such as generating content and translating documents. However, LLMs still fall short of accurately translating literary works, especially given that languages are constantly evolving. The complexities of translating between languages, like English to Chinese or vice versa, cannot be fully captured by simply matching words and phrases.
To address this, developers have created an AI translation team based on the concept of multiagent collaboration. This team includes a CEO, junior and senior editors, translators, proofreaders, and more, all working together. Through multiple rounds of review and refinement, they produce a translation that stays true to the original text while accurately conveying the meaning in the target language.
Though the stability of this method still requires refinement, its potential is already evident, particularly in scenarios that simulate real-world environments where different experts collaborate to achieve a goal.
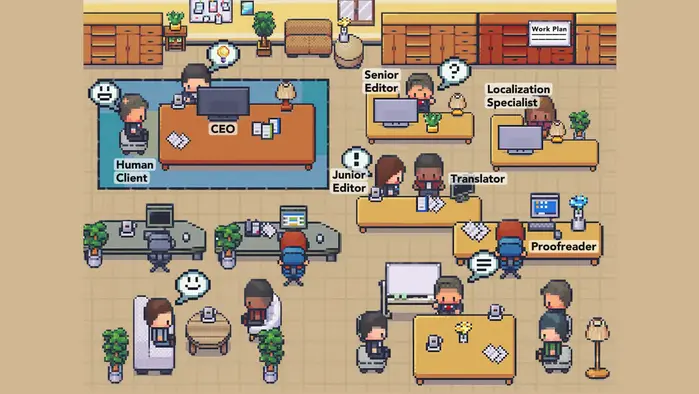
The Future of AI Agentic Workflow
As AI Agentic Workflow continue to evolve, these four design patterns will play increasingly crucial roles in the future of AI development. Andrew Ng reminds us that AI systems using the Agentic Workflow model will take more time to refine their responses or complete their tasks, so patience is essential. Just as a craftsman takes time to perfect their work, or a manager gives an employee time to revise their tasks, we must allow AI agents the time they need to deliver their best results.
AI Agentic Workflows are more than just a progression of current technologies—they represent a foundational shift in the future of AI. This concept moves us beyond single AI systems, ushering in an era where multiple AI systems work together, marking a new chapter in AI’s evolution.
[References]
1 Sequoia Capital. “What’s Next for AI Agentic Workflows Featuring Andrew Ng of AI Fund.” YouTube, 27 March 2024, https://youtu.be/sal78ACtGTc?si=qEhK464ViQCBuXiM.
2 “Machine Translation Goes Agentic.” The Batch, DeepLearning. AI, 15 August 2024, www.deeplearning.ai/the-batch/transagents-a-system-that-boosts-literary-translation-with-a-multi-agent-workflow/.